Black box investments have become increasingly popular in recent years. As the name suggests, they are investment strategies where the internal workings are not fully disclosed to clients. Black box investments typically utilize complex mathematical models and algorithms to make investment decisions. They can be managed by hedge funds, banks, asset management firms etc. Compared to traditional active investments, black box investments promise potential advantages like higher returns, lower fees, and systematic risk management. However, their opacity also leads to risks related to model errors, overfitting, and concentration. This article will analyze the nature, advantages and risks of black box investments in details.
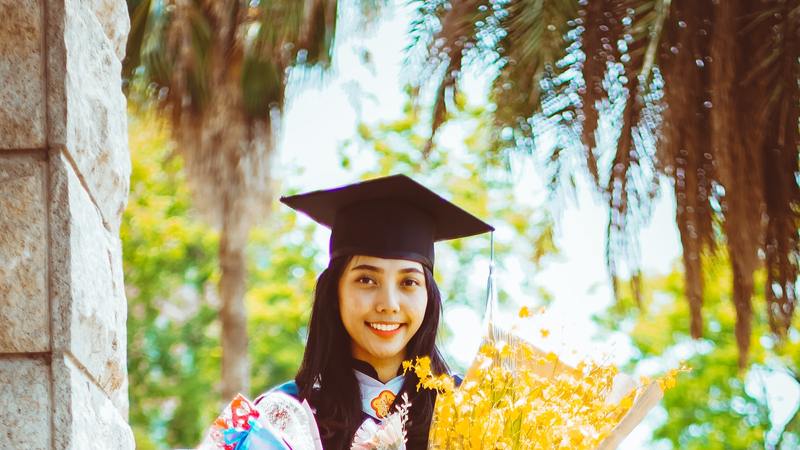
Black box investments rely on quantitative models and algorithms
The defining characteristic of black box investments is their reliance on quantitative models and algorithms to make investment decisions, without disclosing the full details to investors. The models and algorithms are treated as proprietary information by the investment managers. The investment process is automated based on these models. Human discretion is limited once the models are put in place. Typical black box investments include quantitative hedge funds, smart beta ETFs, robo advisors etc. The models input various market data, run complex statistical analysis, and output trading signals or portfolio allocations. Machine learning techniques are increasingly used to develop predictive models. With massive computing power, black box models are able to analyze large amounts of data to identify patterns and opportunities. The models are constantly refined as more data becomes available.
Black box models promise potentials like higher returns and lower fees
Proponents argue black box models have advantages over traditional active investments. Firstly, by eliminating individual biases and emotions, black box models promise to deliver higher risk-adjusted returns. They systematically monitor a huge universe of securities and exploit even minor inefficiencies. Secondly, black box investments have lower management fees because they require less human capital. There is no need for expensive analysts or fund managers. Everything is automated. Thirdly, black box models impose disciplined risk management through pre-defined limits on exposures. Risk can be monitored and controlled in real time. Therefore, black box investments are viewed as the next evolution in asset management. Passive investments provide low-cost exposure to asset classes while black box investments apply algorithms to uncover new return opportunities.
However, the opacity of black box models leads to potential risks
Despite their promised advantages, the opacity of black box models also introduces new risks that investors should be aware of. Firstly, if the models have errors or are overfitted, they will make systematic mistakes repeatedly. Without knowing the logic behind the models, investors cannot assess their robustness. The models may work well in certain periods but fail in new market regimes. Secondly, similar to the risks of overdiversification in passive investments, black box models may end up investing too broadly without real insights. The pursuit of minor efficiencies leads to concentration risks and crowded trades. Thirdly, complex black box models require strong computational power and extensive data to function well. Any technology glitches or data problems can lead to disruptions. Overall, while black box models have strengths, their opacity requires investors to exercise caution and conduct proper due diligence.
In conclusion, black box investments rely on quantitative models and algorithms to deliver potential advantages like higher returns, lower fees, and systematic risk management. However, the opacity of black box models also introduces risks related to potential errors, overfitting, concentration, and technology disruptions. Investors should gain adequate transparency into the investment process, assess the robustness of the models, and implement ongoing oversight.